Information-Maximizing Sampling to Promote Tracking-by-Detection
ICIP'2018, Athens, Greece, Oct 7-Oct 10, 2018
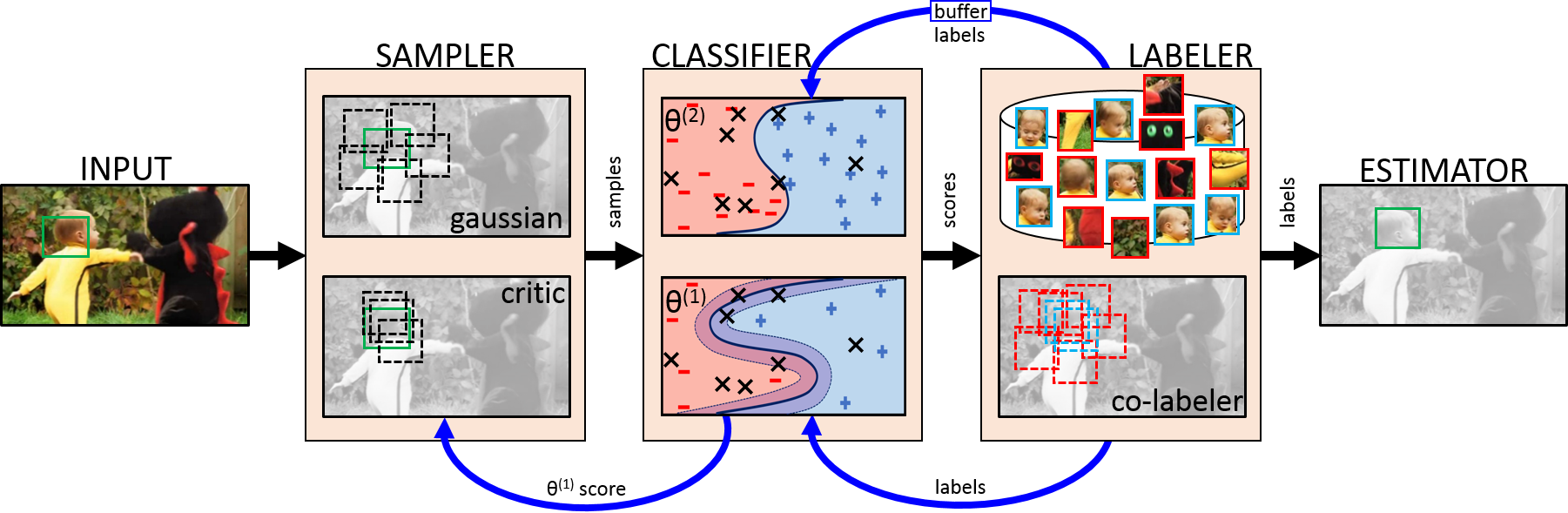
The performance of an adaptive tracking-by-detection algorithm not only depends on the classification and updating processes but also on the sampling. Typically, such trackers select their samples from the vicinity of the last predicted object location, or from its expected location using a predefined motion model, which does not exploit the contents of the samples nor the information provided by the classifier. We introduced the idea of most informative sampling, in which the sampler attempts to select samples that trouble the classifier of a discriminative tracker. We then proposed an active discriminative co-tracker that embed an adversarial sampler to increase its robustness against various tracking challenges. Experiments show that our proposed tracker outperforms state-of-the-art trackers on various benchmark videos.
Overview
To define the region-of-interest for sampling, some tracking-by-detection used context information, optical flow, dynamics model, or a pool of motion models but most of these methods suffers from sudden failures. This is mainly characterized to assumption that the last predicted target location is accurate, an assumption that can be violated under challenging real-world scenarios such as abrupt or fast target movements, occlusions or severe clutters. Object proposal generators, such as Edge boxes, CPMC, and Selective Search are a group of models that provide a fine-selected set of candidates that potentially contain the object in the image. These models, provided that they can provide efficient and reliable candidates can serve as the sampler in a tracking-by-detection pipeline.
In this study, to illustrate the role of sampling in providing better information for the classifier, we address the problem of uninformed sampling by proposing information-maximizing sampling, in which a critic unit learns to sample the essential parts of the image: the most informative ones for the classifier. It is realized by using a semi-supervised co-tracking framework in which the information exchange is managed by active learning, selecting the most uncertain samples of each classifier and querying it from the other. One of the classifiers have a short memory, and the other one enjoys a long memory and updated less frequently, and the critic always challenge the one with short-term memory. Using critic solves uninformed sampling, active learning tackles treat samples unequally, co-learning addresses label noise and together with short-long memory mixture resist model drift.
In frame t the sampler selects three samples A, B, and C to evaluate by the classifier Θ_t. While samples A and B are easy for the classifier to classify, a label for sample C would be uncertain. If Θ_t is used to label sample C, it would be misclassified as positive sample. On the other hand, since this sample is located near the decision boundary of classifier, knowing the correct label of C is crucial to effectively update the model to Θ_t+1. Thus among these, C is the most informative sample (principle of uncertain sampling) and an auxiliary classifier is needed to provide its label (co-learning).
Success Plots
Poster
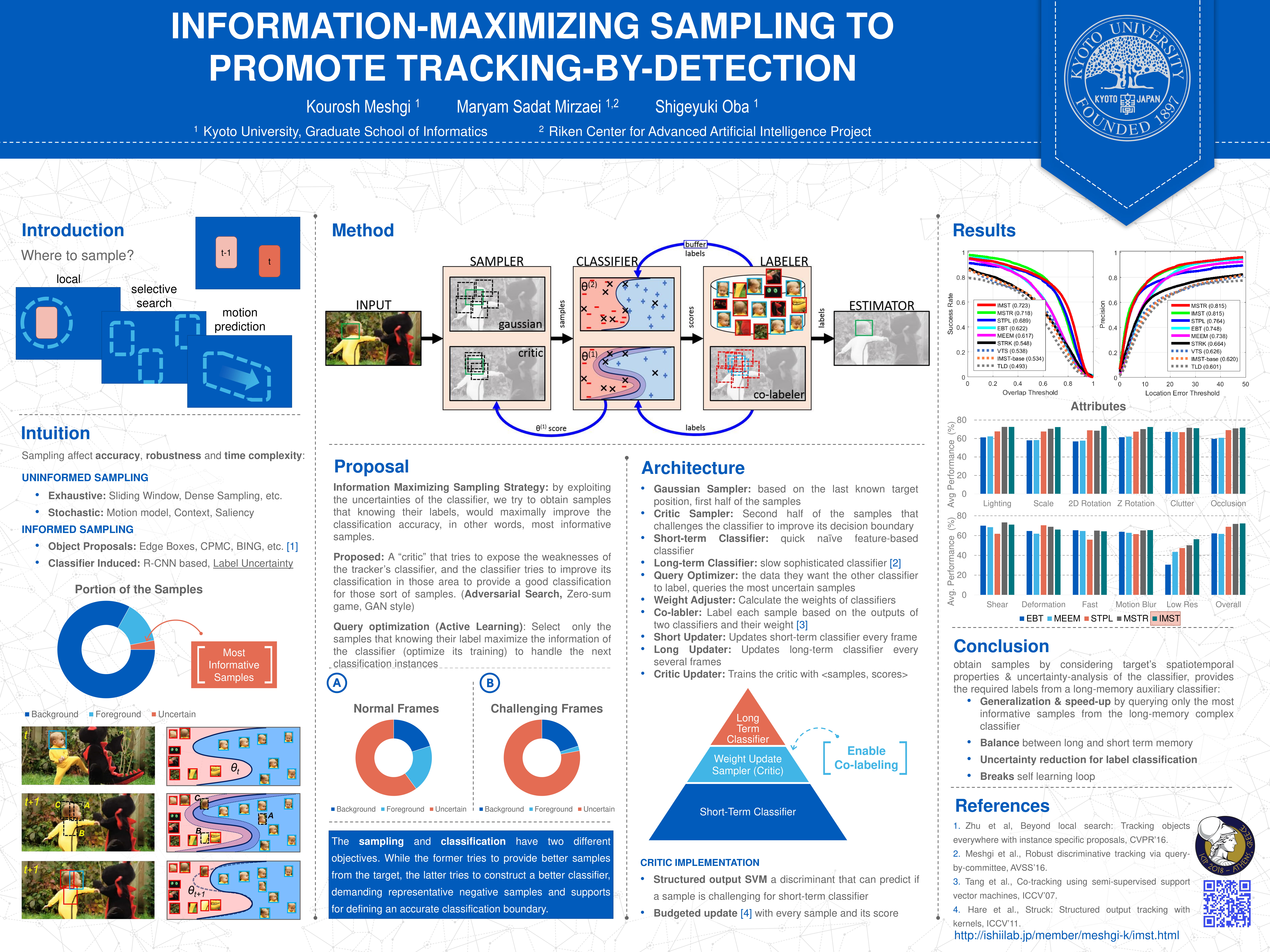
BibTex reference
Download Citation , Google Scholar
@INPROCEEDINGS{meshgi2018imst,
author={Meshgi, Kourosh and Mirzaei, Maryam Sadat and Oba, Shigeyuki},
booktitle={2018 IEEE International Conference on Image Processing (ICIP)},
title={Information-Maximizing Sampling to Promote Tracking-by-Detection},
year={2018},
volume={},
number={},
pages={2700-2704},
doi={10.1109/ICIP.2018.8451725},
ISSN={2381-8549}
}
Acknowledgements
This article is based on results obtained from a project commissioned by the Japan NEDO and was supported by Post-K application development for exploratory challenges from the Japan MEXT.
For more information or help please email meshgi-k [at] sys.i.kyoto-u.ac.jp.