Active Collaborative Ensemble Tracking
IEEE Advanced Video and Signal-based Surveillance (AVSS) 2017, Lecce, Italy, Aug 29-Sep 1, 2017
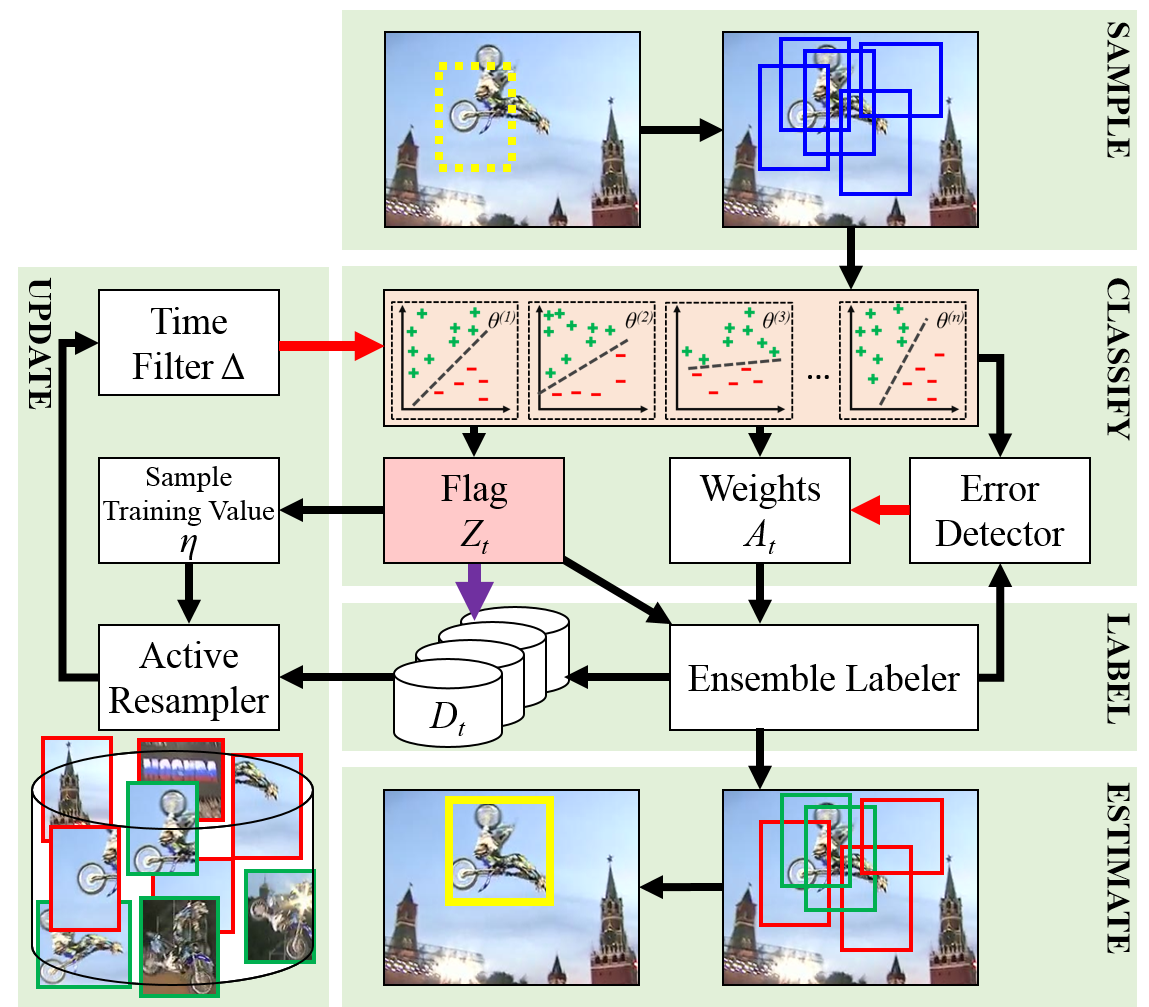
A discriminative ensemble tracker employs multiple classifiers, each of which casts a vote on all of the obtained samples. The votes are then aggregated in an attempt to localize the target object. Such method relies on collective competence and the diversity of the ensemble to approach the target/non-target classification task from different views. However, by updating all of the ensemble using a shared set of samples and their final labels, such diversity is lost or reduced to the diversity provided by the underlying features or internal classifiers' dynamics. Additionally, the classifiers do not exchange information with each other while striving to serve the collective goal, i.e., better classification. In this study, we propose an active collaborative information exchange scheme for ensemble tracking. This, not only orchestrates different classifiers towards a common goal but also provides an intelligent update mechanism to keep the diversity of classifiers and to mitigate the shortcomings of one with the others. The data exchange is optimized with regard to an ensemble uncertainty utility function, and the ensemble is updated via co-training. The evaluations demonstrate promising results realized by the proposed algorithm for the real-world online tracking.
Overview
We propose an effective integration of ensemble tracking and co-tracking, which involves the merits of each while their complementary nature counteracts the demerits of each other. Here, an ensemble of trackers is employed to label a sample. Those classifiers that are uncertain about the label, are excluded from the final decision about the sample's label, and the rest of the classifiers perform a weighted voting for labeling the sample. The contributing classifiers are then retrained with the newly labeled samples, based on the concept of co-training. If the classifiers disagree each other for most of the samples, it is likely that the target is mostly occluded. The use of an ensemble would undermine label noise problem, while co-training breaks the self-learning loop, provides an effective model update, and enforce the diversity into the ensemble. By providing different memory spans for different members of the ensemble, the model update rate of the ensemble is automatically adjusted to the evolution rate of the target, and limited memory horizon resolves the issues with non-stationarity of the observations. By limiting the classifiers' retraining data to only the most informative ones (i.e., to assume different ``training values'' for samples), the non-stationarity is better addressed, the generalizability of the ensemble is improved, and speed of the tracker is boosted.
Success Plots
Other Experiments
Comparison of the proposed tracker (ACET) with online boosting tracker (OAB) that utilizes different features to construct weak classifiers as ensemble members, and randomized ensemble tracker (RET) that make different strong classifiers out of a pool of weak classifiers, and construct the ensemble out of those strong classifiers. We also include BSBT and MIL to represent ensemble trackers based on semi-supervised and multi-instance learning. Here, we implement a version of our tracker (ACET-) that use the same feature set to construct different members of the ensemble and the active learning and memory horizon subsampling is disabled. For the sake of compatibility with published RET results, 13 overlapping sequences with OTB-50 have been used.
Snapshot Video
BibTex reference
Download Citation , Google Scholar
@article{meshgi2017acet,
author={Meshgi, Kourosh and Mirzaei, Maryam Sadat and Oba, Shigeyuki and Ishii, Shin},
booktitle={2017 14th IEEE International Conference on Advanced Video and Signal Based Surveillance (AVSS)},
title={Active collaborative ensemble tracking},
year={2017},
volume={},
number={},
pages={1-6},
doi={10.1109/AVSS.2017.8078534},
month={Aug},
}
Acknowledgements
This article is based on results obtained from a project commissioned by the Japan NEDO and was supported by Post-K application development for exploratory challenges from the Japan MEXT.
For more information or help please email meshgi-k [at] sys.i.kyoto-u.ac.jp.