Computational Cognitive Psychology
Natural environments surrounding humans include unobservable states and dynamically change with time. Even in such complex environments, humans learn the characteristics of the current environment and predict the environmental changes, and it enables them to determine their optimal behaviors. The prediction-based information processing is considered an advanced ability of higher organisms such as humans, and this ability is fundamental to communications. Communication is established by interaction with companion, but his emotional states cannot be directly observed and dynamically change. In such a case, humans predict the emotional states and notions of the companion based on the observable (available) information such as speech, action and expression, and this make for smoother communication. This information processing is achieved by functional brain network.
Brain is the extremely complicated system, both structurally and functionally. However, its structures and functions have been revealed by a great number of psychological and neurophysiological studies. Also in information science field, various computational models on human brain functions are suggested. In our laboratory, we focus on the higher order functions such as learning, language, memory and communication, and study both the model constructions and its verification experiments using a non-invasive brain imaging. We suggest that the integration of the findings from both top-down and bottom-up approach is very important to real understanding of the brain system.
Reinforcement learning in brain
Humans are able to quickly follow the environmental change and make appropriate decisions in consideration of the ambiguity of the environment. Assuming the optimality of the decision-making is defined by a feedback signal given from the environment, the optimal decision-making problem can be solved by reinforcement learning (RL) method.
RL that has been applied to the learning of computers and robots is from animal learning in origin. If a consequence is pleasant, the animals the preceding behavior will become more frequent, and if a consequence is unpleasant the behavior becomes less likely to occur. Voluntary behavior changes based on the results induced by the behaviors, and this association learning between behaviors and rewards is called an operant conditioning in psychology field.
Recent neurophysiological studies indicate that the neuromodulators in the brain: e.g. noradrenaline and serotonin are deeply involved in the dynamical decision-making. Some studies suggested that the learning mechanisms similar to RL algorithms are possibly executed in the brain. Additionally, some imaging studies have suggested that some parts of the RL scheme can be associated with the processing of neural systems in the brain. The RL, originate in the behavioral psychology, develops as the learning theory model in the mathematical and the engineering fields, and be suggested the possibility of the realization in the real brain by the neurophysiological and the cognitive psychology studies.
We focus on the RL method and propose a possible implementation in the brain. In our model, the major parts of the RL scheme are involved in functions of the prefrontal cortex; the dorsolateral prefrontal cortex (DLPF) executes the maintenance and manipulation of the reward-based environmental model, the anterior cingulate cortex (ACC) the action selection depending on the current environmental model and the anterior prefrontal cortex is related to the estimation of unobservable states.
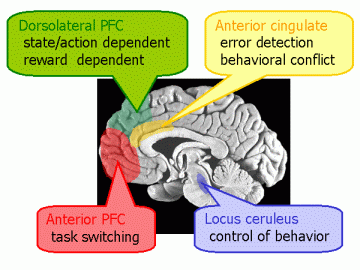
fMRI study
As various brain imaging technologies, such as PET, MRI and MEG, advances, it became to possible to examine the human brain activity closely. Consequently, the studies on human intelligent functions have been make substantial progress.
In order to examine our functional model stated above, we conduct the cognitive psychological study using functional magnetic resonance imaging (fMRI). In the experiments, subjects performed sequential learning tasks on pressing buttons dependently on visual stimuli. An experiment consisted of two conditions; a Markov Decision Process (MDP) condition and a sequential memory condition. By comparing the brain activation between two task conditions, we can identify the brain areas can be considered to have functions that are needed to solve the MDP tasks. Our imaging results is consistent with our hypothesis, in which DLPF is involved in maintenance and manipulation of the environmental model, and ACC is related to action selection.
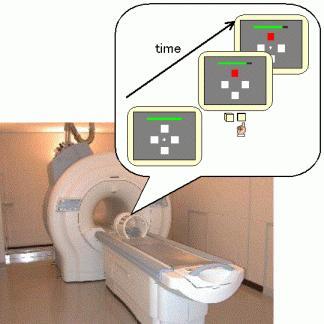
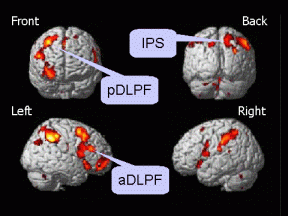